Analytics Uncovered: Deciphering the Terminology of AI
Published:
Dec 6, 2022

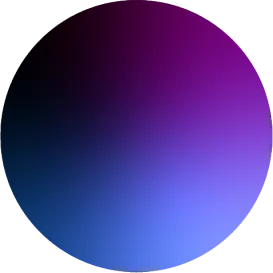

This article originally appeared in the September/October 2022 issue of Security Today as part of their Analytics Uncovered series.
Part 1: Terminology
If you are in the physical security industry, there’s no escaping discussion on video analytics, their advanced capabilities and the barrage of terminology being associated with what promises to be a turning point for physical security and business intelligence applications.
What does it all mean, and where to start?
Here is information on video analytics accessible and easy to understand for all. We will take a high-level look at various aspects of today’s intelligent, AI-driven video analytics. From what analytics really can do, to how they work, to where they can be best applied and even how they differ. The goal is not to be Ph.D.-perfect, but to provide the tools you need to understand the world of analytics. It is down-to-earth, and a no-frills guide for you.
What is New
Before diving in, it is important to understand how analytics got to where it is today.
Traditional programming consists of using data inputs and written programs to provide an output or action. Essentially, software engineers write code to instruct machines how to act. Machines then follow the instructions, repeating the required action over again.
In contrast, Machine Learning is more akin to human learning, and humans learn by patterns, not by simply following instructions. As babies, we recognize that crying leads to receiving food. As adults, we bring an umbrella with us on a cloudy day. We are conditioned to problem-solve based by observing examples of actions and reactions.
The new world of AI-driven video analytics is a pattern recognition on a massive scale. Known actions and their corresponding reactions analyzed by software, and through the process of machine learning, provided with programs that can detect, analyze new and evolving patterns.
A fundamental example is AI-driven video analytics for smoke detection. Computers get images, and videos of smoke (input) and provided with alerting (output). Machine learning creates a program that recognizes smoke, and thus alerts users to its occurrence.
Large-scale pattern recognition is applied at the micro level. Smoke may not be visible to the naked eye, but computers can look at instances of smoke on a pixel-by-pixel level. The ability to use machine learning in this way makes it possible to problem-solve in ways not previously possible by humans. This is the present and future of analytics today.
Terminology, Simplified
There are several key terms in the exploration of AI-driven video analytics. Some we have touched on briefly already and are likely to touch on again. Others you will also see used as marketing buzzwords to make a company sound “innovative” or “advanced,” but are often not used correctly, further contributing to AI-driven video analytics’ mystique.
The following is a glossary of terms designed to give necessary information without the need for a software engineering degree.
Algorithm. Instructions embedded in computer software to convert inputs (questions) to outputs (answers).
Artificial Intelligence. A broad, wide-ranging term to describe the process of making machines and computers capable of performing tasks traditionally relegated to human intelligence.
Computer Vision. Uses video and digital images to extract data for analysis.
Machine Learning. A subset of AI that allows machines to learn from patterns.
Deep Learning. A subset of Machine Learning that seeks to mimic how the human brain works to create patterns and make predictions.
Model. The output of a Machine Learning algorithm that results in pattern recognition. Also known as a program.
Training. The process of sending evidence, or examples of what is necessary, to the computer to create a model.
Inference. The process of putting the model into action to make a prediction.
Understanding Deep Learning
Deep Learning is perhaps the most advanced subset of AI. Since it attempts to mimic the human brain, it far surpasses the brain’s capabilities – and it does so via Neural Networks.
Imagine you are viewing a blurry scene. Add a lens like when undergoing an eye exam, and the scene becomes clearer. Add another lens, and even more definition is visible. The more lenses added, the sharper and more detailed is the image. This system of adding layers (lenses in this example) to progressively understand a 'scene' is similar to how we think about modern Machine Learning.
Each additional lens becomes its own individual Neural Network. When multiple lenses are added, it is called a Convolutional Neural Network (CNN). Deep Learning is when Machine Layers layers added, each building upon the previous layer to create better patterns to a more complex problem. For example, one Machine Layer may detect a vehicle, the next layer detects a license plate, and the final layer reads the license plate. Each of these layered models can combine to form a Deep Learning Model capable of solving a more complex vehicle identification challenge.
Related Content
Book a Meeting
Discover the Power of Dragonfruit
Schedule a meeting to experience the future of enterprise video analytics with Dragonfruit AI's cutting-edge technology.
What to expect
We'll be in touch shortly
Submit the form and we'll make sure the right person is in touch as soon as possible.
We'll set up a Personal demo
We'll identify the right solutions to fit your needs and show how it can work for you.
We'll customize a custom plan
We'll create a plan catered to your business to help drive impact quickly.
