What Drives Accuracy in Video AI? Key Factors Behind Reliable Outcomes
Published:
Jan 31, 2025
.png)
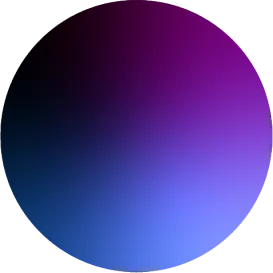
.png)
In the world of video AI, accuracy isn’t just a feature. It’s the foundation. With industries increasingly relying on AI for tasks ranging from surveillance to customer behavior analysis, the stakes are higher than ever. But with so much data to process, how do we ensure these video intelligence solutions stay reliable and precise?
Key Factors Influencing Accuracy
Achieving high accuracy in video AI solutions requires a combination of hardware, data quality, model training advanced algorithms, and real-time processing capabilities. In a landscape where the demand for precision is growing rapidly, understanding the core factors that influence AI performance is crucial. These factors are what allow AI to distinguish meaningful patterns from noise, ensuring that the insights generated are both relevant and actionable. Let’s take a look!
Quality of Input Data
The foundation of any effective video AI solution lies in the quality of its input data. High-resolution video feeds (ideally 720p or higher) are essential for accurate object detection and tracking. Additionally, well-placed cameras that minimize obstructions and distortions can significantly enhance data quality. Factors such as lighting conditions also play a crucial role as low-light environments can hinder performance unless advanced low-light algorithms are employed.
Algorithmic Architecture
The effectiveness of video AI systems relies heavily on the algorithms they use. Modern systems often use deep learning models, like Convolutional Neural Networks (CNNs), to spot and identify objects in video frames. These models can be enhanced with other techniques to improve accuracy. For example, Recurrent Neural Networks (RNNs) help the system understand changes over time. This is called temporal analysis, which allows the AI to track moving objects or recognize patterns that develop across multiple video frames.
Additionally, Transformer models can help the AI understand the bigger picture by considering the context of what’s happening. The combination and configuration of these algorithms can make a big difference in how well the system performs.
Domain-Specific Training
Training data must be tailored to the specific application of the video AI solution. For instance, a solution designed for retail analytics should be trained on datasets that reflect typical customer interactions and behaviors in retail environments. It's not just about the video itself, but also about including extra details or annotations, like customer demographics or the placement of products on shelves. Moreover, as things change over time—like shopping habits or store layouts—continuous updates to training datasets ensure that models remain relevant and effective in such changing environments.
Real-Time Processing Capabilities
In many applications, especially in security and surveillance, real-time processing is crucial. The ability to analyze video feeds with minimal latency ensures timely alerts and responses to potential incidents. To that end, edge processing has become a game changer. By processing data closer to the source—on devices like cameras or local servers—edge processing reduces latency, minimizes bandwidth usage, and allows for faster decision-making. This means that video AI systems can react in real time, even in environments with limited internet connectivity or when dealing with large amounts of data.
READ: Top AI Video Surveillance Trends to Watch in 2025: Technologies Shaping the Future of Security
Contextual Understanding
True accuracy in video AI goes beyond simple object detection; it requires an understanding of context. For instance, identifying suspicious behavior in a retail setting requires more than just spotting an individual acting oddly at a particular moment. It involves analyzing behavior over time and recognizing patterns that deviate from what’s normal for that specific environment.
Advanced video AI solutions go beyond basic detection by integrating spatial reasoning, which maps observed behaviors against the physical layout of the environment, like the layout of a store or the positioning of key areas (e.g., entrances, aisles, checkout counters). Additionally, these systems employ temporal analysis, allowing them to track and compare activities across multiple timeframes. This makes it possible to spot anomalies that wouldn’t be obvious from a single frame of video, such as someone lingering too long in a restricted area or moving in a fashion deviating from typical customer behavior.
By combining spatial and temporal understanding, these solutions can better identify potential threats or opportunities, delivering more accurate and context-aware insights.
Validation and Testing Rigor
To ensure that video AI systems stay accurate, they need to undergo thorough testing at every stage. This helps make sure the system works correctly in real-world situations. One common method is A/B testing, where the AI solution is tested against older or existing systems to see if it performs better in detecting and analyzing data. This allows developers to compare the new system’s performance with something they know works, helping them identify improvements.
Another key technique is confusion matrix analysis. This is used to evaluate how well the system identifies and classifies objects or actions. For example, it helps check whether the system is correctly identifying a person or mistaking them for something else. It measures precision (how accurate the system is when it makes a prediction) and recall (how many of the correct predictions the system actually detects). The goal is to reduce errors and make sure the system recognizes what it's supposed to without missing important details.
READ: How Video AI Reduces False Alarms and Improves Risk Management
Adversarial testing is another important step. This tests how well the solution can handle attempts to deceive or "trick" it. For example, a system used for security might be tricked by someone wearing a mask, so adversarial testing ensures the system is tough enough to handle these situations and continue working reliably.
Lastly, continuous monitoring is crucial. Once the AI solution is in use, it must be regularly checked to make sure it keeps performing well and make timely adjustments to algorithms based on real-world feedback.
Human-in-the-Loop Feedback
Incorporating human oversight into AI workflows can significantly enhance accuracy. Human operators can review low-confidence predictions flagged by the system, providing corrections that improve future model performance. This collaborative approach ensures that the AI system learns from its mistakes, refining its predictions over time.
Unlock Reliable Video AI Performance with Dragonfruit AI
The demand for higher accuracy in AI-powered video solutions is not just a trend, it’s a necessity. Whether in security, retail, or operational intelligence, businesses need solutions that minimize errors and maximize actionable insights.
Dragonfruit AI is leading the charge by delivering AI-driven video solutions where accuracy is the foundation—not an afterthought. By prioritizing precision across applications, we help businesses make informed decisions that drive security, efficiency, and profitability.
READ: Better, Cheaper & More Powerful: The Best AI Apps for Your Business
We leverage a multi-layered approach to ensure its AI-driven video solutions deliver best-in-class accuracy:
- AI-Powered Object Recognition: Our solutions use deep learning models to accurately differentiate between humans, vehicles, and background elements, reducing misclassification and enhancing decision-making.
- Contextual & Real-Time Analysis: Unlike rule-based systems, our AI understands movement context, minimizing false positives and focusing on real security threats.
- Continuous Model Improvement: With our Kaizen Data Flywheel, Dragonfruit AI continuously refines its models based on real-world data, ensuring the system stays up to date.
- Scalable, Cost-Effective Processing: Using our patented Split AI architecture, we process data closer to the source, reducing latency while maintaining the high accuracy of cloud-based processing.
- Customer-Specific Adaptation: Dragonfruit AI learns from your unique environment, enhancing accuracy with tailored solutions and
- Human-in-the-Loop Feedback for continuous refinement.
Want to see AI-driven accuracy in action? Contact us today!
Let’s Connect.
Related Content
Book a Meeting
Discover the Power of Dragonfruit
Schedule a meeting to experience the future of enterprise video analytics with Dragonfruit AI's cutting-edge technology.
What to expect
We'll be in touch shortly
Submit the form and we'll make sure the right person is in touch as soon as possible.
We'll set up a Personal demo
We'll identify the right solutions to fit your needs and show how it can work for you.
We'll customize a custom plan
We'll create a plan catered to your business to help drive impact quickly.
